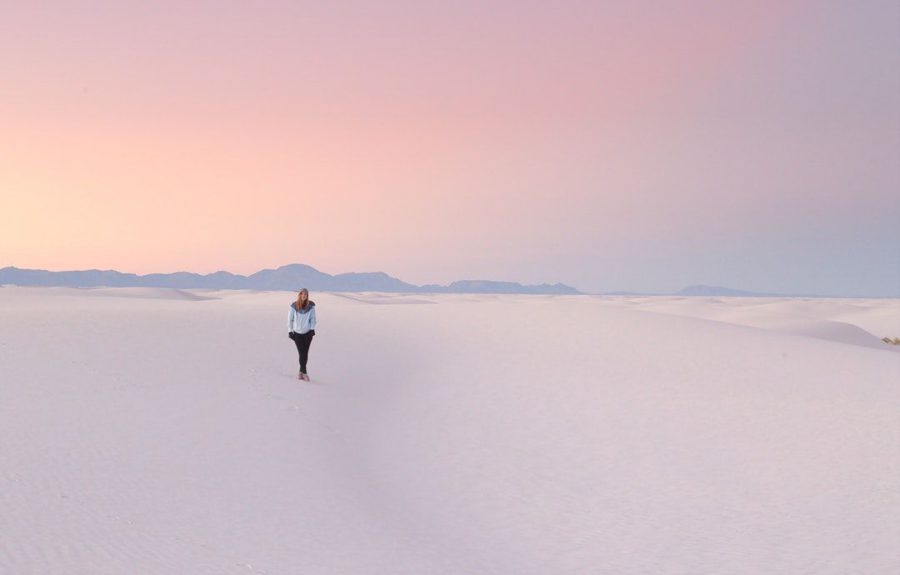
Arvind Narayanan, Joanna Huey and Ed Felten have published a paper on the potential for large datasets to be used to identify individuals.They argue that groups releasing data should ‘stop relying on [ad hoc de-identification methods]…as a sufficient privacy protection on its own’ because this makes it impossible to know whether people might be identified through data analysis.
What’s their solution? They say that, at the moment, if an organisation releasing data has used ‘ad hoc’ de-identification methods and taken out information that could identify individuals (personally identifiable information or PII):
the burden of proof falls on privacy advocates to show that the particular datasets are re-identifiable or could cause other harms.
Instead, they suggest that the group releasing data should be required to show that the data does not allow people to be identified or cause harms – a so-called ‘precautionary’ approach. (This doesn’t mean that should be no risk at all, but that it should be balanced against the benefits of releasing the data). Is this realistic for advocacy organisations? Let us know in the comments.
Open government data
They also examine five specific cases for this principle, suggesting that ‘the problem of “what to do about re-identification” unravels once we stop looking for a one-size-fits-all solution’.
Perhaps the most useful for the responsible data community is the case for releasing open data, where, as they say, ‘in most cases there is no ability to opt out of data collection.’ They then assess the risks involved:
- Re-identification worries are minimal because the vast majority of open government datasets do not consist of longitudinal observations of individuals.
- For a variety of datasets ranging from consumer complaints to broadband performance measurement, the data is not intended to track users longitudinally, but it might accidentally enable such tracking if there is enough information about the user in each measurement data point.
- Certain aggregate or low-dimensional government data, such as many of the datasets published by the U.S. Census Bureau, seem to avoid privacy violations fairly well by using statistical disclosure control methodologies.
- However, high-dimensional data [which contains many individual data points for each individual record] is problematic, and there is no reason to expect it cannot be de-anonymized.
The authors then recommend that any group releasing open data implements provable privacy techniques like differential privacy, or wait ‘until provable privacy techniques can be implemented satisfactorily’.
Is this a feasible approach for people using data in the service of social good objectives, who aren’t always familiar with managing large data sets? The Responsible Data Forum is hosting a 90-minute online discussion to introduce basic de-identification strategies, such as perturbation, trimming and psuedonomynization. A small group of practitioners will discuss their limitations, the contexts in which they’re most appropriate, and the expertise and tools required to use them. Join discussion and planning on this and other events: http://lists.theengineroom.org/lists/info/responsible_data.
Thanks for sharing this. The Precautionary Principle took a hard hit in the early days of the GMOs debates as it was critiqued for being unimplementable. More recently it has been given new life by Nassim Nicholas Taleb who has taken a more mathematical, risk-management approach to its analysis and implementation. For more on this, read his paper or listen to this excellent interview on Econtalk. Taleb makes the critical distinction between scale-dependent and scale-independent harm. A car crash is a scale-dependent harm. The damage is limited to the vehicles involved in the collision. It doesn’t suddenly cause all cars to crash. A decision by a car manufacturer to remove the brakes from its vehicles is an example (albeit ridiculous) of a scale independent harm. That decision will affect all the cars made by that manufacturer as well as any other cars sharing the road with cars made by that manufacturer. Taleb argues that GMOs incur a scale-independent risk. I think the concept of scale-dependence or independence is an invaluable insight when considering risk.
The frictionless and boundless environment of the Internet represents another kind of scale-independent risk. When privacy is violated on the Internet, it is an all or nothing scenario. Either no one knows your private information or everyone can know it. The leak of celebrity photos from Apple is a case in point. Thus I really welcome this paper which attempts to set out some principles about how the precautionary principle might be applied in several different data collection contexts. The last context they deal with is that of Open Government Data (OGD) and they waffle a bit on it. They acknowledge that much OGD has low privacy concerns but they also point out that many data resources, which have no intention of revealing longitudinal patterns about data subjects, may do so inadvertently. They also point out that high-dimensional OGD resources are vulnerable to de-identification.
In reading through this and reflecting on the Open Data principles embraced by many governments of which a prominent principle is “Open By Default”, I am grappling with the question of whether a responsible approach to OGD can simply be open by default or whether a more precautionary approach which involves more risk analysis upfront is called for. Perhaps one might get creative and apply something like what the South African Sustainable Seafood Initiative and apply a green, amber, or red tag to different types of government datasets that would call for varying levels of “precaution” prior to publication. I would love to hear others’ thoughts as for me this is an unresolved tension.